ISCB-Asia/SCCG 2012 Keynote Address
Prof. Eric Xing
Carnegie Mellon University
Reverse Engineering Evolving Gene Networks Underlying Developing Biological Systems: a principled statistical machine learning approach
|
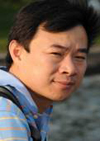
|
Abstract
Estimating rewiring gene regulatory networks over developing biological systems, such as proliferating cells, growing embryos, and differentiating cell lineages, is central to a deeper understanding of how cells evolve during development. However, one challenge in estimating such evolving networks is that their host cells are not only contiguously evolving, but also branching over time. For example, stem cells evolve into two more specialized daughter cells at each division, forming a tree of networks. Another example is in a laboratory setting: a biologist may apply several different drugs to a malignant cancer cell to analyze the changes each drug has produced in the treated cells. Each treated cell is not directly related to another treated cell, but rather to the malignant cancer cell that it was derived from.
We propose a novel statistical framework, which builds on the L1 plus total variation penalized graphical logistic regression, to effectively estimate multiple evolving gene networks corresponding to cell types related by either a linear-sequence or a tree-genealogy, based on only a few samples from each cell type. Our method takes advantage of the similarity between related networks along the biological lineage, while at the same time exposing sharp differences between the networks. We demonstrate that our method performs significantly better than existing methods via simulation, and enjoys strong statistical guarantees unlike other heuristic based approaches. Furthermore we explore an application to a breast cancer analysis. Based on only a few microarray measurements, our algorithm is able to produce biologically valid results that provide insight into the progression and reversion of breast cancer.
Biography
Dr. Eric Xing is an associate professor in the School of Computer Science at Carnegie Mellon University. His principal research interests lie in the development of machine learning and statistical methodology; especially for solving problems involving automated learning, reasoning, and decision-making in high-dimensional, multimodal, and dynamic possible worlds in social and biological systems. Professor Xing received a Ph.D. in Molecular Biology from Rutgers University, and another Ph.D. in Computer Science from UC Berkeley. His current work involves, 1) foundations of statistical learning, including theory and algorithms for estimating time/space varying-coefficient models, sparse structured input/output models, and nonparametric Bayesian models; 2) computational and statistical analysis of gene regulation, genetic variation, and disease associations; and 3) large-scale information & intelligent system in social networks, computer vision, and natural language processing. Professor Xing has published over 160 peer-reviewed papers, and is an associate editor of the Annals of Applied Statistics (AOAS), the Journal of American Statistical Association (JASA), the IEEE Transaction of Pattern Analysis and Machine Intelligence (PAMI), the PLoS Journal of Computational Biology, an Action Editor of the Machine Learning journal, and a member of the DARPA Information Science and Technology (ISAT) Advisory Group. He is a recipient of the NSF Career Award, the Alfred P. Sloan Fellowship, the United States Air Force Young Investigator Award, the IBM Open Collaborative Research Award, and best paper awards in a number of premier conferences including UAI, ACL, SDM, and ISMB.